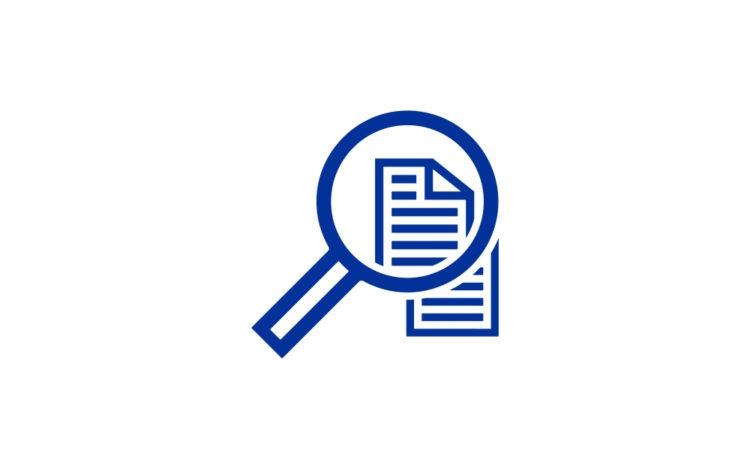
Recent advances in artificial intelligence (AI) have revived the debate about the impact of new technologies on jobs (e.g. Frey and Osborne, 2017; Susskind, 2020; and Acemoglu, 2021). Waves of innovation have usually been accompanied by anxiety about the future of jobs. This apprehension persists, even though history suggests that previous fears about labour becoming redundant were exaggerated (e.g. Autor, 2015; Bessen, 2019). In fact, in this article we show that during the deep learning boom of the 2010s, occupations potentially more exposed to AI-enabled technologies actually increased their employment share in Europe. However, the jury is still out on whether the same can be expected from new developments in AI-enabled technologies. When the verdict comes in, it could be win or lose – not only for jobs, but also for equality and prosperity in Europe.
The evidence so far on AI and employment
AI breakthroughs have come in many fields. These include advances in robotics, supervised and unsupervised learning, natural language processing, machine translation and image recognition, to name but a few. AI has applications among masses of other activities that enable automation of human labour in non-routine tasks, both in manufacturing and also in services – from providing medical advice to writing code. AI is thus a general-purpose technology that can automate work in virtually every occupation. It stands in contrast to other technologies such as computerisation and industrial robotics, which only allow a limited set of tasks to be automated by implementing manually specified rules.
The empirical evidence on the effect of AI-enabled technologies on jobs and wages is still evolving. For example, both Felten et al. (2019) and Acemoglu et al. (2022) conclude that occupations more exposed to AI experience no significant impact on employment. However, Acemoglu et al. (2022) also find that AI-exposed establishments reduced non-AI and overall hiring. That would imply that while new tasks are being created, AI is also replacing human labour in a subset of tasks. Moreover, Felten et al. (2019) find that occupations impacted by AI actually experience a small rise in wages. On a different note, Webb (2020) argues that, compared with either software or robots, AI-enabled technologies are likely to have a greater impact on high-skilled workers in particular. Meanwhile, the literature to date focuses mostly on the United States.
New evidence for Europe
In a recent paper (Albanesi et al. 2023), we examine the link between AI-enabled technologies and employment shares in 16 European countries over the period 2011-19[2]. These years saw the rise of deep learning applications such as language processing, image recognition, algorithm-based recommendations or fraud detection. Though more limited in scope than the current generative AI models such as ChatGPT, deep learning applications are nonetheless revolutionary – and still trigger concerns about the impact on jobs. We use data at three-digit occupation level (according to the International Standard Classification of Occupations) from Eurostat’s Labour Force Survey, as well as two proxies for potential AI-enabled automation borrowed from the literature. The first proxy is the AI Occupational Impact created by Felten et al. (2018) and Felten et al. (2019), which links advances in specific applications of AI to abilities required for each occupation as described in O*NET[3]. The second one is a measure of the exposure of tasks and occupations to AI, constructed by Webb (2020) by quantifying the text overlap between AI patent descriptions and job descriptions from O*NET. In addition, we compare the exposure to AI-enabled technologies with exposure to software by using a software exposure measured as constructed by Webb (2020).
Chart 1
Exposure to technology by education level
average percentile
Source: Albanesi et al. (2023). Notes: The chart reflects how exposed different “education groups” of workers are on average to the three technology measures. Education groups are defined as the subsample of occupation-sector cells whose average educational attainment is in the lower, middle, and upper third (tercile) respectively of the national education distribution.
What do the results say about the impact of AI on employment?
We find a positive association between AI-enabled automation and changes in employment shares in our sample of 16 European countries, regardless of which proxy we used. According to the AI exposure indicator proposed by Webb (2020), moving 25 centiles up along the distribution of exposure to AI is associated with a 2.6% increase in sector-occupation employment share, while using the measure provided by Felten et al. (2018, 2019) the estimated increase in the sector-occupation employment share is 4.3%. The estimated coefficients are displayed by the horizontal line in the left-hand and middle columns of Charts 2 and 3.
Technology-enabled automation might also affect the relative shares of employment at different skill levels and thus impact earnings inequality. The literature on job polarisation shows that medium-skilled workers in routine-intensive jobs tended to be replaced by computerisation (e.g. Autor et al, 2003; Goos et al, 2009). In contrast, it is often argued that AI-enabled automation is more likely to complement or replace jobs in occupations that employ high-skilled labour.
Panels (a) and (b) in Chart 2 show the estimated coefficients of the association between changes in employment and AI-enabled automation by level of education (broken down into terciles, i.e. the lower, middle and upper thirds of the population). Statistically significant coefficients are plotted in dark blue. For occupations where average educational attainment is in the low and medium-skill groups, AI exposure does not seem to shake things up significantly. However, for the high-skill group we find a positive and significant association: moving 25 centiles up along the distribution of exposure to AI appears to boost the sector-occupation employment share by 3.1% using Webb’s AI exposure indicator, and by 6.7% using the measure of Felten et al.
Chart 2
Exposure to technology and changes in employment shares by skill level
a) AI, Webb b) AI, Felten et al. c) Software, Webb
percentage changes
Source: Albanesi et al. (2023). Notes: Regression coefficients measuring the effect of exposure to technology on changes in employment share. Each observation is a ISCO 3-digit occupation times sector cell. Observations are weighted by cells’ average labour supply. Sector and country dummies are included. The sample consists in data for 16 European countries, from 2011 to 2019. The coefficient for the whole sample is shown by the horizontal line. The bars display the coefficient estimated for the subsample of cells for average educational attainment in the lower, middle and upper tercile respectively of the within-country education distribution. Coefficients that are statistically significant at least at the 10% level are plotted in dark blue.
Panels (a) and (b) in Chart 3 report the estimates by age group (again broken down by terciles, i.e. youngest, middle and oldest third of the population). AI-enabled automation appears to be more favourable for those occupations that employ relatively younger workers. Regardless of the AI indicator used, the estimated benefit for the younger group seems to be double that for the other age groups.
AI-enabled automation is thus associated with employment increases in Europe – mostly for high-skill occupations and younger workers. This is at odds with the evidence from previous technology waves, when computerisation decreased the relative share of employment of medium-skilled workers, resulting in polarisation. However, we do not find evidence of this polarisation pattern for our sample, even when examining the impact of software-enabled automation, proxied by the software exposure by Webb (2020). Panel (c) in Charts 2 and 3 displays the results. The relationship between software exposure and employment changes is null for the pooled sample, and there is no evidence of software replacing routine medium-skill jobs.
Chart 3
Exposure to technology and changes in employment shares by age
a) AI, Webb b) AI, Felten et al. C) Software, Webb
percentage changes
Source: Albanesi et al. (2023). Notes: Regression coefficients measuring the effect of exposure to technology on changes in employment share. Each observation is an ISCO 3-digit occupation times sector cell. Observations are weighted by cells’ average labour supply. Sector and country dummies are included. The sample consists in data for 16 European countries, from 2011 to 2019. The coefficient for the whole sample is shown by the horizontal line. The bars display the coefficient estimated for the subsample of cells of workers with an average age in the lower, middle and upper tercile respectively of workers’ age distribution. Coefficients that are statistically significant at least at the 10% level are plotted in dark blue.
Despite the results for employment shares, neither AI or software exposure showed statistically significant effects on wages, except when using the Felten et al. measure, which indicates that occupations more exposed to AI have slightly worse wage growth.
Our results show a mixed picture across the 16 European countries. The positive impact of AI-enabled automation on employment holds true for most countries, with only a few exceptions. However, the scale of the impact varies substantially across countries. This might reflect differences in underlying economic factors, such as the pace of technology diffusion and education, or the level of regulation – and therefore competition – in product and labour markets.
Too soon to reach a verdict
During the deep learning boom of the 2010s, occupations potentially more exposed to AI-enabled technologies actually increased their employment share in Europe. Occupations with a relatively higher proportion of younger and skilled workers gained the most. For wages, the evidence is less clear and suggests neutral to slightly negative impacts. These results do not amount to an acquittal: AI-enabled technologies continue to be developed and adopted. Most of their impact on employment and wages – and therefore on growth and equality – has yet to be seen.
References
Acemoglu, D (2022), “Harms of AI”, Oxford Handbook of AI Governance.
Acemoglu, D., Autor, D., Hazell, J. and Restrepo, P. (2022), “Artificial intelligence and jobs: Evidence from online vacancies”, Journal of Labor Economics, Vol. 40(S1), pp. S293-S340.
Acemoglu, D. and Restrepo, P. (2018), “The race between man and machine: implications of technology for growth, factor shares, and employment”, American Economic Review, Vol. 108, pp. 1488-1542.
Albanesi, S., Dias da Silva, A., Jimeno, J. F., Lamo, A. and Wabitsch, A. (2023), “New Technologies and Jobs in Europe”, Working Paper Series, No 2831, ECB.
Autor, D. (2015), “Why are there still so many jobs? The history and future of workplace automation”, Journal of Economic Perspectives, Vol.29(3), pp. 3-30.
Autor, D., Levy, F. and Murnane, R. (2003), “The skill content of recent technological change: An empirical exploration”, Quarterly Journal of Economics, Vol. 118(4), pp. 1279-1333.
Bessen, J. (2019), “Automation and jobs: when technology boosts employment” , Economic Policy, October, pp. 589-626.
Felten, E. W., Raj, M. and Seamans, R. (2018), “A method to link advances in artificial intelligence to occupational abilities”, AEA Papers and Proceedings, Vol. 108, pp. 54–57.
Felten, E. W., Raj, M. and Seamans, R. (2019), “The effect of artificial intelligence on human labor: An ability-based approach”, Academy of Management Proceedings.
Frey, C. B. and Osborne M. A. (2017), “The future of employment: How susceptible are jobs to computerisation?”, Technological Forecasting and Social Change, Vol. 114, pp. 254-280.
Goos, M., Manning, A. and Salomons, A. (2009), “Job polarization in Europe”, American Economic Review, Vol. 99(2), pp. 58-63.
Susskind, D. (2020), A world without work: Technology, automation and how we should respond, London: Penguin.
Webb, M. (2020), The impact of artificial intelligence on the labor market, mimeo.